Storage architectures must change to meet the needs of AI – and Huawei thinks it has a head start on competitors
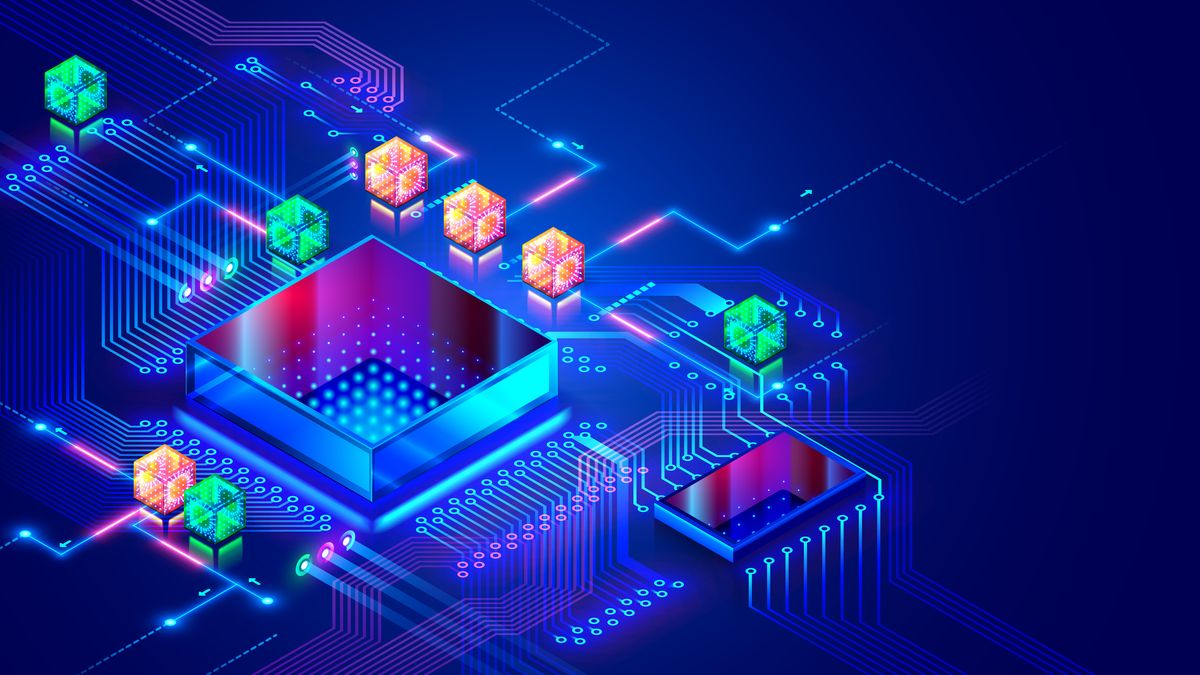
The ongoing AI boom has precipitated a change in computational methods from the CPU to GPU, and storage architectures will need to adapt to meet the new demands of AI workloads, according to a Huawei exec.
As illustrated by the unprecedented rise of Nvidia to become the AI chipmaker par excellence, the industry has transposed much of its computation demands on GPU data centers to train their AI models.
Peter Zhou, president of data storage product line at Huawei, thinks there needs to be a corresponding change in storage systems to accommodate the needs of these GPU-based facilities.
Speaking to ITPro, Zhou explained how the storage industry has remained relatively stagnant for the last decade, but advancements in machine learning, generative AI, and big data will continue to disrupt the space and force vendors to innovate.
“There were not really any big changes happening in that domain , the industry like EMC or NetApp, they have been doing what they have been doing for 10 years. Their product has not really been changed for about 10 years, but when big data and AI came to reality, everything changed” he explained.
“First of all the value of data changed…Data is not just used for recording, data becomes an asset that machines can use to study [the world], it’s the source of their knowledge and can enable their ‘thinking’, and now the data storage industry has to be changed”.
The IT industry, therefore, has to focus on ensuring their storage solutions can both accommodate the new volume of data being collected and ensure it is made available at the speeds these systems require.
Data mobility is storage industry’s biggest challenge
Zhou detailed how storage has a vital role to play in driving the efficiency of these GPU-based computation systems, noting that key considerations such as energy consumption and performance are vital for the modern business engaging in AI development.
“In today’s GPU the work efficiency is less than about 50%, which means that half the time the GPU is just waiting there and wasting energy. The only way to change this is by making the data storage system more efficient, and then the efficiency of these GPUs can be improved by about 30%, which is a lot of energy.”
But to achieve the level of efficiency these GPU clusters need, the underlying architecture of storage systems needs to evolve, according to Zhou.
“I think the architecture of data storage has to be changed. Today, out of the box data storage, the architecture is exactly the same as the normal CPU-centric architecture, which is very old,” he noted.
“The CPU is there, there’s memory directly connecting to the memory, the memory is so small and the data cannot be stored in the memory long term. And then they use the I/O interface to connect all these disks, and then when the CPU is processing the data, this data needs to be brought to the memory, and then and then back to the processor etc.”
Zhou stated roughly 60% of energy consumed by these storage devices is used for data mobility, and reducing this is the challenge storage vendors will need to overcome in coming years.
Huawei’s in-house chipset capabilities could be its greatest strength for storage innovation
The challenge for the industry, in Zhou’s view, is that the hardware needs to be changed to meet these new efficiency requirements, but the major players in data storage aren’t designing or producing their own chipsets.
This consigns them to using general purpose CPUs and other components, leaving potential efficiency gains on the table – and this is where Zhou argued Huawei have an advantage due to their in-house chip production.
“We are designing and producing our own chipsets, which means we have the capability to change the architecture of the computational system.”
He ran through some changes the company has made to move to what he describes as a ‘data-centric’ architecture. Earlier this year, for example, Huawei announced it would move away from I/O interfacing with its storage architectures with all of its components now connected to a universal data bus, leveraging Intel’s Compute Express Link (CXL) to enable faster communication with the GPU.
Zhou also raised decoupling the data plane from the control plane in storage systems, providing benefits in terms of the scalability, performance, flexibility, and management, thereby helping to avoid performance bottlenecks when tasked with large amounts of data and particularly complex AI workloads.
Many of these changes follow similar adaptations Nvidia has made on the computational side of the equation, Zhou said, adding that storage architectures will inevitably need to follow its example to adapt to the evolving needs of the industry.
“If you look into the Nvidia GPU cards, you can sense the architecture of the computational system is changing. This means data storage has to be changed to reflect this.”
Source link